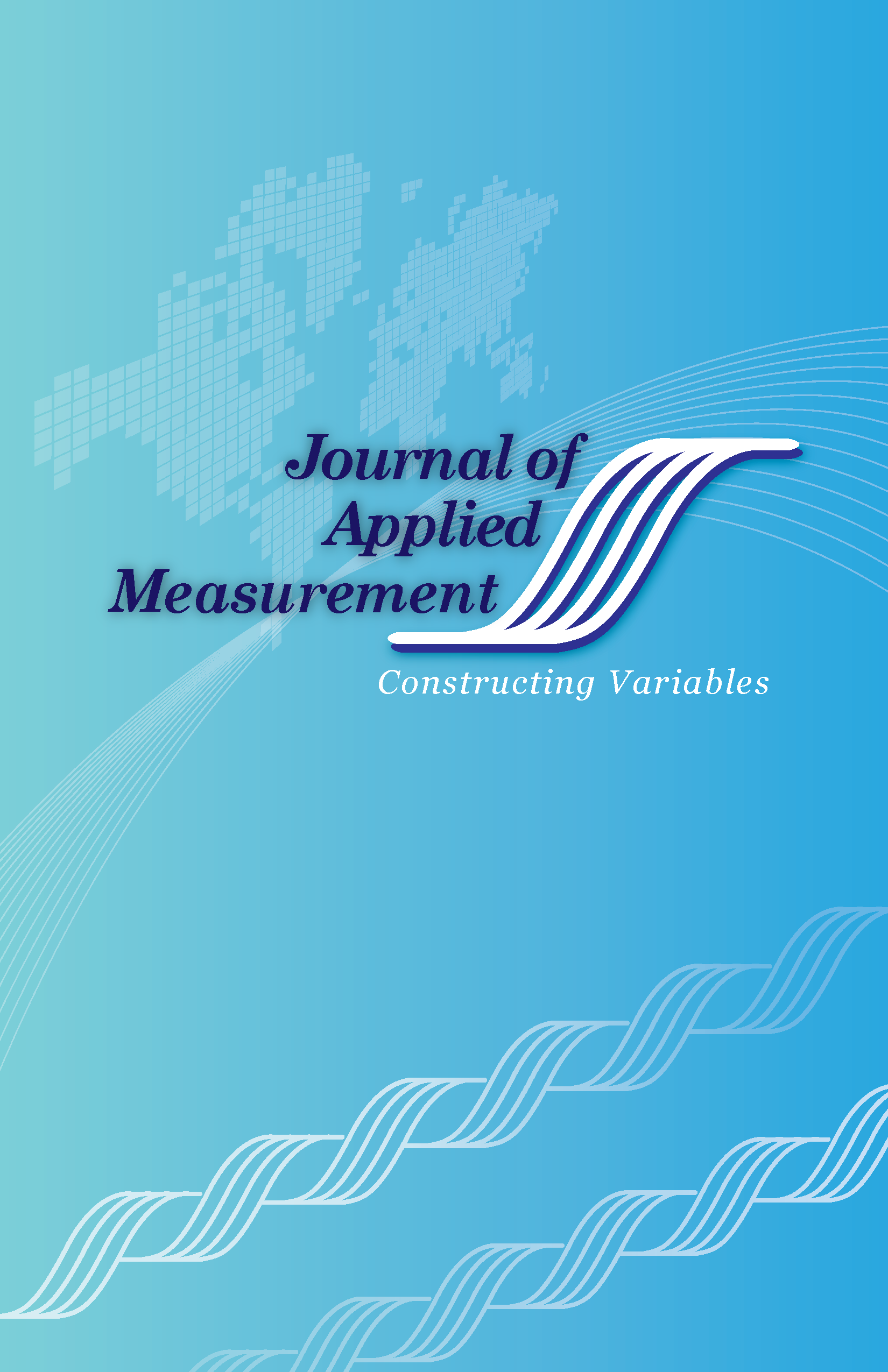
Journal of Applied Measurement
A publication of the Department of Educational Psychology and Counseling
National Taiwan Normal University
Volume 24, Issue 3/4 (2023)
Editorial: Reflection on the Role of AI in Measurement and Assessment
Hak Ping Tam
National Taiwan Normal University
n/a
Citation:
Tam, H. P. (2023). Editorial: Reflection on the Role of AI in Measurement and Assessment.. Journal of Applied Measurement, 24(3/4), i–iv.
Using Explanatory Item Response Models to Evaluate Surveys
Jing Li
University of Georgia
George Engelhard
University of Georgia
This study evaluates the psychometric quality of surveys by using explanatory item response models. The specific focus is on how item properties can be used to improve the meaning and usefulness of survey results. The study uses a food insecurity survey (HFSSM) as a case study. The case study examines 500 households with data collected between 2012 and 2014 in the United States. Eleven items from the HFSSM are classified in terms of two item properties: referent (household, adult, and child) and content (worry, ate less, cut meal size, hungry, and not eat for the whole day). A set of explanatory linear logistic Rasch models is used to explore the relationships between these item properties and their locations on the food insecurity scale. The results suggest that both the referent and item content are significant predictors of item location on the food insecurity scale. It is demonstrated that the explanatory item response models are a potential method for examining the psychometric quality of surveys. Explanatory item response models can be used to enhance the meaning and usefulness of survey results by providing insights into the relationship between item properties and survey responses. This approach can help researchers improve the psychometric quality of surveys and ensure that they are measuring what they intend to measure. It can lead to better-informed policy decisions and interventions aimed at tackling social issues such as food insecurity, poverty, and inequality.
Keywords: Explanatory item response models, linear logistic Rasch model, household food insecurity, surveys
Citation:
Li, J., & Engelhard, G. (2023). Using explanatory item response models to evaluate surveys. Journal of Applied Measurement, 24(3/4), 88–101.
Psychometric Properties of the Statistical Anxiety Scale and the Current Statistics Self-Efficacy Using Rasch Analysis in a Sample of Community College Students
Samantha Estrada Aguilera
University of Texas at Tyler
Emily Barena
University of Texas at Tyler
Erica Martinez
University of Texas at Tyler
Community college students have rarely been the focus of study within statistical education research. This study aims to examine the psychometric properties of two popular scales utilized within statistics education: Current Statistics Self-Efficacy (CSSE) and Statistical Anxiety Scale (SAS) focusing on a population of community college students. A survey was conducted on N = 161 community college students enrolled in an introductory statistics course. The unidimensional structure of the CSSE was confirmed utilizing a confirmatory factor analysis (CFA), and after selecting the rating scale model approach, we found no misfitting items and good reliability. Concurrent and discriminant validity was examined utilizing the SAS. The SAS three-factor structure was also assessed, examining the item fit. We found that an item in the SAS subscale, Fear of Asking for Help, was flagged as misfitting. Overall, both the CSSE and SAS demonstrated sound psychometric properties when utilized with a population of community college students.
Keywords: Rasch analysis, statistical anxiety, statistics self-efficacy
Citation:
Estrada, S., Barena, E., & Martinez, E. (2023). Psychometric properties of the Statistical Anxiety Scale and the Current Statistics Self-Efficacy using Rasch analysis in a sample of community college students. Journal of Applied Measurement, 24(3/4), 102–120.
Modeling the Effect of Reading Item Clarity on Item Discrimination
Paul Montuoro
The University of Western Australia
Stephen Humphry
The University of Western Australia
The logistic measurement function (LMF) satisfies Rasch’s criteria for measurement while allowing for varying discrimination among sets of items. Previous research has shown how the LMF can be applied in test equating. This article demonstrates the advantages of dividing reading test items into three sets and subsequently applying the LMF instead of the Rasch model. The first objective is to examine the effect of item clarity and transparency on item discrimination using a new technique for dividing reading items into sets, referred to as an item clarity review. In this article, the technique is used to divide items in a reading test with different levels of discrimination into three sets. The second objective is to show that, where three such sets exist, the subsequent application of the LMF leads to improved item fit compared to the standard Rasch model and the subsequent retention of more items. The item sets were shown to have different between-set discrimination but relatively uniform within-set discrimination. The results show that, in this context, reading test item clarity and transparency affect item discrimination. These findings and other implications are discussed.
Keywords: logistic measurement function, Rasch, item clarity review
Citation:
Montuoro, P., & Humphry, S. (2023). Modeling the effect of reading item clarity on item discrimination. Journal of Applied Measurement, 24(3/4), 121–132.
Differences in School Leaders’ and Teachers’ Perceptions on School Emphasis on Academic Success: An Exploratory Comparative Study
Sijia Zhang
University of North Carolina
Cheng Hua
University of Montevallo
This quantitative study examined how principals and teachers from all participating countries and regions perceive school emphasis on academic success (SEAS) differently. Participants (N = 26,302) were all principals and teachers who filled out the SEAS scale from PIRLS 2021. A second-order confirmatory factor analysis and a many-faceted Rasch analysis were used to investigate the psychometric properties of the SEAS and whether there existed differences in school leaders’ and teachers’ perceptions of such construct within and across countries. Results from the factor analysis yielded a three-factor solution, and the SEAS scale demonstrated satisfying psychometric properties. Rasch analysis indicated great model-data fit, and item-level fit statistics. Future studies are encouraged to explore the psychometric properties of SEAS and how SEAS impacts other school-related variables and student outcomes. This study explored a new instrument to measure academic emphasis and compared leaders’ and teachers’ perceptions of SEAS in an international setting.
Keywords: school emphasis on academic success, principal and teacher, psychometric properties, Rasch analysis, PIRLS 2021
Citation:
Zhang, S., & Hua, C. (2023). Differences in school leaders’ and teachers’ perceptions on school emphasis on academic success: An exploratory comparative study. Journal of Applied Measurement, 24(3/4), 133–149.
Analysis of Multidimensional Forced-Choice Items Using Rasch Ipsative Models With ConQuest
Xuelan Qiu
Institute for Learning Sciences & Teacher Education, Faculty of Education and Arts, Australian Catholic
University
Dan Cloney
Australian Council for Educational Research
Multidimensional forced-choice (MFC) items have been widely used to assess career interests, values, and personality to prevent response biases. This tutorial first introduces the typical types of MFC items and the item response theory models to analyze MFC items. It further shows how to analyze the dichotomously and polytomously scored MFC items with paired statements based on the Rasch ipsative models using the computer program ACER ConQuest. The assessment of differential statement functioning using the ConQuest was also demonstrated.
Keywords: multidimensional forced-choice, item response theory, model estimation, differential statement functioning, test fairness
Citation:
Qiu, X., & Cloney, D. (2023). Analysis of multidimensional forced-choice items using Rasch ipsative models with ConQuest. Journal of Applied Measurement, 24(3/4), 150–170.